AI in financial fraud detection: uncovering insights
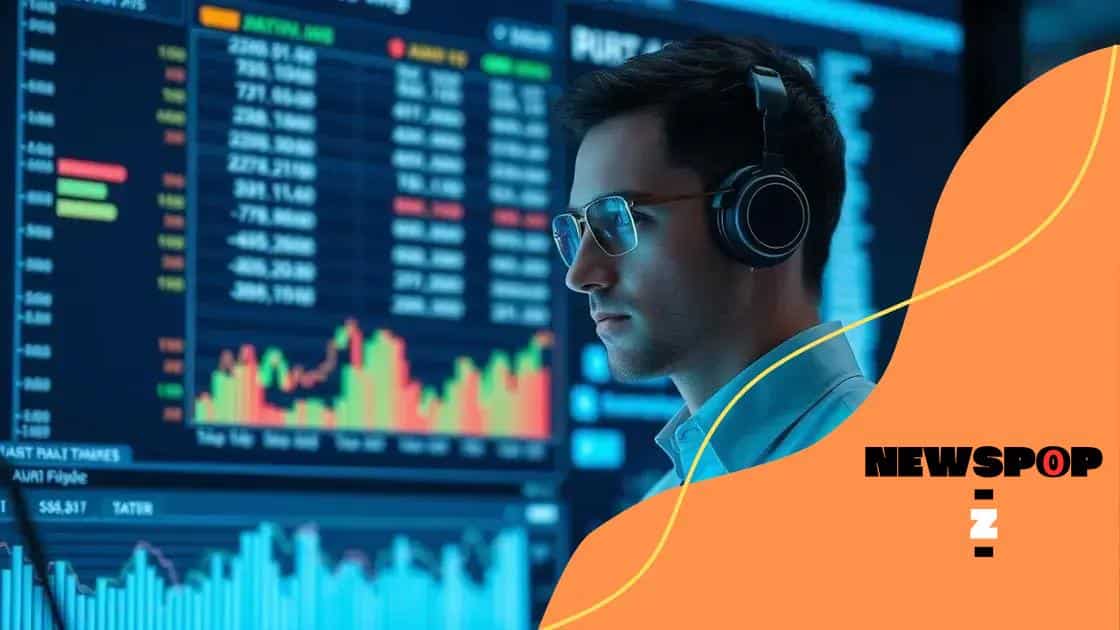
AI in financial fraud detection utilizes advanced algorithms and big data to identify suspicious patterns, enhance security measures, and provide real-time monitoring, making it essential for protecting organizations against fraudulent activities.
AI in financial fraud detection is changing the game for businesses. Have you ever wondered how machine learning algorithms can identify fraud? Let’s dive into this innovative technology and explore its impact.
Understanding financial fraud
Understanding financial fraud is crucial for protecting businesses and consumers alike. It involves various methods that criminals use to illegally gain money or property. Awareness of these methods can help organizations implement better defenses.
Types of Financial Fraud
Financial fraud comes in many forms. Here are some common types:
- Credit Card Fraud: Unauthorized use of a credit card to purchase goods or services.
- Investment Fraud: Scams that promise high returns with little risk to investors.
- Insurance Fraud: False claims made to receive benefits unjustly.
- Identity Theft: Stealing personal information to commit fraud.
Each type has its own unique characteristics, making it essential for organizations to know how to identify them. Financial fraud can also involve more sophisticated techniques, such as using technology to fake documents or siphon data from secure systems.
Common Red Flags
It’s important to recognize the signs of potential fraud. Look for:
- Unusual Account Activity: Sudden changes in transaction patterns.
- Lack of Documentation: Missing training records or correspondence.
- Poor Internal Controls: Weak procedures for verifying transactions.
Understanding these red flags can empower organizations to take action before significant losses occur. AI in financial fraud detection enhances the ability to spot these irregularities automatically, streamlining the monitoring process.
As technology evolves, so do the techniques used by fraudsters. Staying informed about the latest trends in fraud is essential. Organizations can benefit from training their employees to recognize fraud attempts and implement best practices.
Implementing effective measures involves investing in robust systems and processes. Organizations should regularly review their anti-fraud strategies to adapt to new threats in the environment.
How AI detects suspicious patterns
AI plays a vital role in identifying suspicious patterns in financial transactions. By using advanced algorithms, it can analyze vast amounts of data quickly and efficiently. This capability significantly reduces the time needed to detect fraud.
Machine Learning Techniques
One of the ways AI detects fraud is through machine learning. This technology learns from historical data to recognize what constitutes normal behavior. Over time, it improves its accuracy in identifying anomalies.
- Supervised Learning: This approach uses labeled datasets to train the model, helping it learn how to differentiate between normal and fraudulent transactions.
- Unsupervised Learning: In contrast, this technique identifies patterns in unlabelled data, making it useful for spotting new or unknown fraud schemes.
- Deep Learning: A subset of machine learning, deep learning mimics the human brain to process data, enabling even more sophisticated pattern recognition.
These techniques allow AI systems to become increasingly effective at spotting trends that a human might miss. By analyzing various factors such as transaction amounts, frequency, and user location, AI can pinpoint suspicious activity.
Real-time Monitoring
Another critical advantage of AI in fraud detection is real-time monitoring. Instead of relying solely on periodic audits, AI continuously scans transactions as they occur. This approach allows organizations to act immediately on potential threats, reducing losses.
Using automated rules combined with AI insights, businesses can flag unusual transactions for further investigation. These flags can be based on multiple criteria, ensuring a comprehensive review of each case.
Integrating AI into existing financial systems enhances security measures. By leveraging data analytics, organizations can adapt their strategies to evolving fraud tactics and protect themselves more effectively.
Real-world applications of AI in finance
There are many real-world applications of AI in finance. Institutions such as banks, investment firms, and insurance companies are leveraging AI technologies to improve efficiency and enhance customer experience.
Fraud Detection
One of the most notable applications is in fraud detection. Financial organizations use AI to analyze transaction data and identify suspicious patterns. By using machine learning algorithms, these systems can quickly adapt to new fraud tactics.
- Pattern Recognition: AI can spot anomalies in transaction data that may indicate fraud.
- Real-time Alerts: Systems can provide immediate alerts for questionable activities, allowing prompt investigations.
- Reduced False Positives: AI helps in minimizing false alarms, allowing staff to focus on genuine threats.
This approach not only enhances security but also saves time and resources significantly.
Customer Service
AI is also revolutionizing customer service in finance. Chatbots and virtual assistants are available 24/7 to answer client inquiries. This technology ensures that customers receive quick, accurate responses without long wait times.
These AI systems can handle multiple requests simultaneously, providing a personalized experience. By analyzing customer interactions, AI can anticipate needs and offer tailored solutions.
Risk Management
Furthermore, AI aids in risk management by offering predictive analytics. Financial institutions assess risk profiles using AI algorithms that process vast amounts of data to forecast potential market movements.
- Credit Scoring: AI evaluates creditworthiness more accurately by analyzing diverse data points.
- Investment Strategies: Algorithms can identify profitable investment opportunities by analyzing market trends.
- Regulatory Compliance: AI technologies can help organizations stay compliant by monitoring transactions against regulations.
Incorporating AI into their operations enables finance companies to make smarter, data-driven decisions, ultimately leading to better outcomes for their clients.
Challenges in implementing AI solutions
Implementing AI solutions in finance presents several challenges. Organizations must navigate technical, ethical, and operational hurdles to reap the benefits of AI technology.
Data Quality and Availability
One significant challenge is the quality of data. For AI to function effectively, it requires high-quality, clean data. However, many organizations struggle with:
- Data Silos: Information is often stored in different systems, making it hard to access.
- Inconsistencies: Variations in data formats can lead to errors in analysis.
- Lack of Comprehensive Datasets: Insufficient data can hinder AI training, resulting in ineffective algorithms.
Improving data quality is essential for successful AI integration.
Integration with Existing Systems
Another challenge is integrating AI solutions with existing financial systems. Many legacy systems are not designed to work with modern AI technologies, leading to:
- Compatibility Issues: Older software may lack the necessary infrastructure for AI implementation.
- High Costs: Upgrading systems can be expensive and time-consuming.
- Disruption: Introducing new technologies can disrupt current operations.
Effective planning and strategic investments are necessary to overcome these integration challenges.
Ethical Considerations
Ethical concerns also arise with the use of AI in finance. Issues regarding bias in algorithms can lead to unfair treatment of customers. Organizations must address:
- Algorithm Transparency: Understanding how AI makes decisions is critical for trust and accountability.
- Data Privacy: Protecting sensitive customer information is essential to maintain compliance and consumer trust.
- Bias and Fairness: Ensuring algorithms are free from bias to protect vulnerable populations.
Developing ethical AI practices is crucial for long-term success.
Overcoming these challenges requires collaboration across departments, investment in employee training, and a commitment to continuous improvement. Organizations that tackle these issues head-on will be well-positioned to leverage AI and gain a competitive advantage.
Future trends in AI and fraud detection
The future of AI in fraud detection is exciting and holds great potential. As technology evolves, we can expect significant advancements that will enhance the effectiveness of fraud prevention measures.
Enhanced Algorithms
One trend is the development of more sophisticated algorithms. These algorithms will increasingly incorporate natural language processing and deep learning. This advancement will allow systems to understand context better, leading to improved accuracy in detecting fraudulent activities.
- Predictive Analytics: AI can anticipate fraudulent actions by analyzing past data and identifying patterns.
- Adaptive Learning: Systems will learn from new data continuously, adjusting their detection methods to remain effective.
- Multi-layered Models: Combining various models will lead to more robust detection capabilities.
These enhancements will improve the overall accuracy of fraud detection systems.
Integration of Big Data
Another key trend is the integration of big data in AI fraud detection systems. With access to vast amounts of data from various sources, AI can analyze even the smallest nuances.
Organizations will be able to combine data from various channels, including social media, transaction records, and market trends. This comprehensive approach will provide deeper insights into customer behavior and potential fraud indicators. As a result, it will lead to more informed decision-making.
Collaboration with Blockchain
Additionally, more companies are exploring the use of blockchain technology to enhance security. Blockchain can provide transparent and tamper-proof records of transactions, making it an invaluable resource in fraud detection.
- Immutable Records: Changes to blockchain records are nearly impossible, providing security against alterations.
- Instant Verification: Transactions can be verified in real-time, reducing the chances of fraud.
- Trustworthy Data Sharing: Organizations can share data securely, enhancing collaborative fraud detection efforts.
As AI collaborates with such technologies, we can anticipate even greater strides in fraud prevention. Overall, these trends will lead to more effective, responsive, and secure systems for detecting fraud.
FAQ – Frequently Asked Questions about AI in Financial Fraud Detection
How does AI help in detecting financial fraud?
AI analyzes large amounts of transaction data to identify suspicious patterns and anomalies that may indicate fraud.
What are common challenges in implementing AI solutions?
Common challenges include data quality issues, integration with existing systems, and ethical concerns regarding bias.
What role does big data play in AI fraud detection?
Big data allows AI systems to analyze diverse data sources, improving the accuracy and effectiveness of fraud detection efforts.
Will blockchain technology enhance fraud prevention?
Yes, blockchain provides secure and transparent transaction records, making it difficult for fraudsters to alter or manipulate data.